A primer on AI

"AI is the new electricity.”
- Dr. Andrew Ng - Founder of DeepLearning.AI, Founder & CEO of Landing AI, General Partner at AI Fund, Chairman and Co-Founder of Coursera and an Adjunct Professor at Stanford University’s Computer Science Department.
Introduction
On November 30th, 2022, ChatGPT was released to the world. ChatGPT, a large language model (LLM) developed by Open AI, quickly went viral in days, crossing over a million users within a week of its launch. The growth is unprecedented; it took 10 months for Facebook to reach a million users and 24 months for Twitter to hit the same metric, whereas ChatGPT arrived at a million users in just 5 days. The reviews were glowing, with many technology enthusiasts likening the experience to using an iPhone or a web browser for the first time. While AI was certainly a vertical of interest to most VCs, with ChatGPT’s launch, AI was quickly accelerated to the forefront of the VC and technology ecosystems. While AI is a rapidly evolving field, it’s a field that has its origins dating back to the early 1950s, when the very first AI programs were developed. Over the past several decades, AI has quickly progressed and advanced, now being used in a wide range of diverse applications. Today, AI is being used across a swath of fields such as healthcare, finance, and transportation (to name a few), and its potential use cases in the future are vast and varied. In this piece, we will explore the history of AI, the current applications of AI, and some of the future uses and developments that are likely to occur in the field, as well as some possible dangers.
Ancient Origins of AI
The history of AI has philosophical roots, which to the surprise of many, actually go back to ancient times. AI has been a topic of philosophical debate and contemplation for centuries, with some of the earliest recorded discussions of the subject dating all the way back to ancient Greece, where AI was seen as a field of study that focused on the creation of machines that could mimic human behavior and thought processes. Some of the earliest works discussing the creation of intelligent machines can be found in the writings of Aristotle and Plato. In his book "Metaphysics," Aristotle described a thought experiment in which a device called a "pneumatical statue" was created. This device used air pressure to move its limbs and imitate the movements of a living being. Aristotle also discussed the concept of a "thinking machine" in his book "On the Soul," in which he speculated about the possibility of creating a machine that could think and reason like a human. Plato, in his book "The Republic," also discussed the concept of intelligent machines. In one passage, he describes a group of people who are so enamored with their own creations that they begin to worship them as gods.
Modern AI
Over time, the term "artificial intelligence" has come to be used more broadly to refer to a wide range of technologies and techniques that are designed to create intelligent machines. This includes not only mathematical algorithms and computational models, but also machine learning, deep learning, natural language processing, and many other technologies. The term "artificial intelligence" (AI) was first coined by John McCarthy, an American computer and cognitive scientist, in 1955. McCarthy, who is often referred to as the "father of AI," defined AI as "the science and engineering of making intelligent machines." The term was intended to capture the idea of creating machines that could think and reason like human beings. At the time, McCarthy and other pioneers in the field of AI were focused on developing mathematical algorithms and computational models that could mimic human intelligence and behavior.
John McCarthy organized The Dartmouth Summer Research Project on Artificial Intelligence, also known as the Dartmouth Conference, which is widely regarded as a seminal event in the history of artificial intelligence. The conference, held at Dartmouth College in the summer of 1956, brought together many of the leading figures in the field of AI at the time to brainstorm ideas for future research and development. These figures included prominent scientists including Marvin Minksy (co-founder of MIT’s AI Lab), Donald MacKay (best known for his work around brain organization and the similarities between computers and the human brain), Ray Solmonoff (the inventor of algorithmic probability), and many more. During the conference, the attendees discussed a wide range of topics related to AI, including the nature of intelligence, the capabilities and limitations of machines, and the ethical implications of creating intelligent machines. The conference also resulted in the creation of influential AI research programs and initiatives, such as the Logic Theorist, which was one of the first AI programs to be able to solve complex problems using logical reasoning.
As the field of AI began to grow and attract more attention, government organizations started to take a keen interest in it. In the United States, for example, the Defense Advanced Research Projects Agency (DARPA) played a key role in supporting critical AI research and development in the 1950s and 1960s, with DARPA funding many of the earliest AI research projects that helped propel the field. In recent years, the role of government organizations in the field of AI has continued to grow. Governments around the world have begun to invest heavily in AI research and development, and have established dedicated agencies or programs to support the growth of the field. For example, the European Union has established the European Commission on Artificial Intelligence, and the Chinese government has launched the Next Generation Artificial Intelligence Development Plan.
In the 1980s, AI research shifted focus towards the development of machine learning algorithms, which are designed to improve their performance on a specific task through experience. This led to the creation of learning systems, including neural networks and decision trees, which have since become a mainstay of modern AI technology.
In recent years, the field of AI has continued to advance at an incredible pace, thanks in part to the vast amounts of data that are now available for researchers to use. This has led to the creation of new AI technologies, such as deep learning algorithms and natural language processing systems, which are capable of performing a wide range of tasks with impressive accuracy.
To briefly summarize the modern history of AI:
- Early 1950s: The field of AI is founded, and the first AI programs are developed.
- 1956: The Dartmouth Summer Research Project on Artificial Intelligence, also known as the Dartmouth Conference, is held.
- 1950s and 1960s: AI research focuses on developing algorithms and computational models that can mimic human intelligence and behavior.
- 1970s: Expert systems, which can mimic the decision-making abilities of human experts, are developed.
- 1980s and 1990s: The field of AI experiences a period of slower progress and funding cuts.
- 1980s: The field of machine learning, which focuses on developing algorithms that can learn from data (machine learning), emerges.
- 1990s: AI research begins to focus on the development of more powerful and efficient computational systems.
- 2000s and 2010s: AI experiences a resurgence, thanks in part to the rise of deep learning, which allows machines to learn complex patterns and relationships from data.
- 2010s: AI technologies become more widespread and are used in a variety of fields, including healthcare, finance, and transportation.
- 2020s: AI continues to advance and becomes an increasingly important part of many industries and applications.
Understanding Modern AI
When discussing AI, many get the following terms confused and commingled: Artificial intelligence (AI), machine learning (ML), deep learning (DL), and neural networks (NN). While all are related topics, they remain distinct fields of study and technology. AI is the broader field of study that encompasses subfields such as those of machine learning, deep learning, and neural networks. Machine learning is a subfield of AI that focuses on developing algorithms and models that allow machines to learn from data. Deep learning is a type of machine learning that uses neural networks to learn from data, and neural networks are computational models that are inspired by the structure and function of the human brain.
- Artificial intelligence (AI) is the broader field of study that deals with the creation of intelligent machines. AI systems are designed to mimic human intelligence and behavior, such as the ability to reason, learn, and make decisions.
- Machine learning (ML) is a subfield of AI that focuses on the development of algorithms and models that allow machines to learn and improve their performance on a specific task over time, without being explicitly programmed.
- Deep learning (DL) is a type of machine learning that uses artificial neural networks to learn and make predictions. These neural networks are designed to mimic the structure and function of the human brain, and they can be trained on large datasets to learn complex patterns and relationships.
- Neural networks (NN) are computational models that are inspired by the structure and function of the human brain. They consist of many interconnected nodes, or "neurons," which can process and transmit information. Neural networks are commonly used in deep learning algorithms to make predictions and learn from data.
AI today tackles a host of problems, covering a swath of industries. Building an efficient AI system is model-based, and is rooted in first-principles thinking. A modern AI developer, after defining a specific problem, gathers a large dataset from which the system can learn from and refine itself. After the dataset has been gathered and scrubbed to ensure cleanliness, the model gets trained to find patterns within the data. Continuous and rigorous evaluation and refinement allows for the model to become better over time, and once the model is ready, it will ultimately get integrated into an application or production environment so that it can make predictions or decisions in real time, solving the problem it was designed for. Today, there are many programming languages that are commonly used for building AI applications. The choice of programming language, however, varies, and often depends on the specific requirements and goals of the AI project, as well as the preferences and expertise of the developers on the project. Some of the prominent AI programming languages in use today include Python, Java, R, Lisp, and Prolog.
Modern AI Use Cases
The use cases for AI are constantly evolving and expanding at lighting speed as the technology continues to advance. AI usage can be seen in a vast array of industries and verticals, many of which are easily recognizable. AI can be used for image and speech recognition, natural language processing, and predictive modeling. AI’s uses are countless and plentiful. AI is put to use in Amazon’s Alexa and Apple’s Siri, can be seen in Tesla’s strides in autonomous vehicles, is used by Spotify and Netflix in their recommender algorithms, and can be seen all across industries such as supply chain management, healthcare, insurance, and manufacturing. Other use cases, however, are not as obvious and tangible. These include education, community-building, content creation, influencer marketing, and data analysis.
Education
AI can be harnessed in education to individualize the learning experience, grade assignments, create adaptive learning systems, analyze student performance data, and much more. These applications, amongst others, not only improve the efficiency and effectiveness of education, but can provide students with an enhanced learning experience. ProfJim, a BGV portfolio company, represents the potential disruptive applications being developed in the edtech space that are propelled by AI. ProfJim, developed by Dr. Deepak Sekar (who holds over 200+ patents himself) developed his own patented AI model that turns otherwise dull textbook PDFs into engaging powerpoint video lectures, narrated by aviators of either famous historical figures or contemporary teachers, such as Aristotle (shown below):
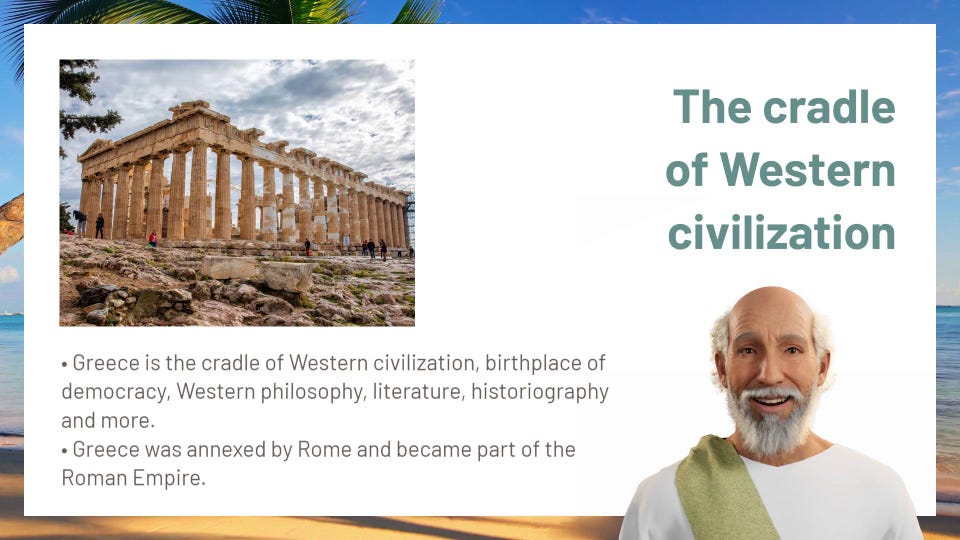
Community-Building
AI has strong potential to enhance the often overlooked vertical of community-building, where it can have a demonstrable impact. AI can impact the community-building experience by serving as a tool that makes it easier to connect with others, facilitates communication and understanding, and creates a more potent and engaging experience for communities by accurately suggesting connections or groups within the community. Intros, an AI-based application that BGV made an investment in within Fund I, does exactly the latter, for example. Intros empowers community builders to create meaningful connections between their members at scale by utilizing AI-generated personalized introductions. Intros unlocks the power of connection within communities, leveraging data and tailored algorithms to make meaningful and worthy interactions within various groups.
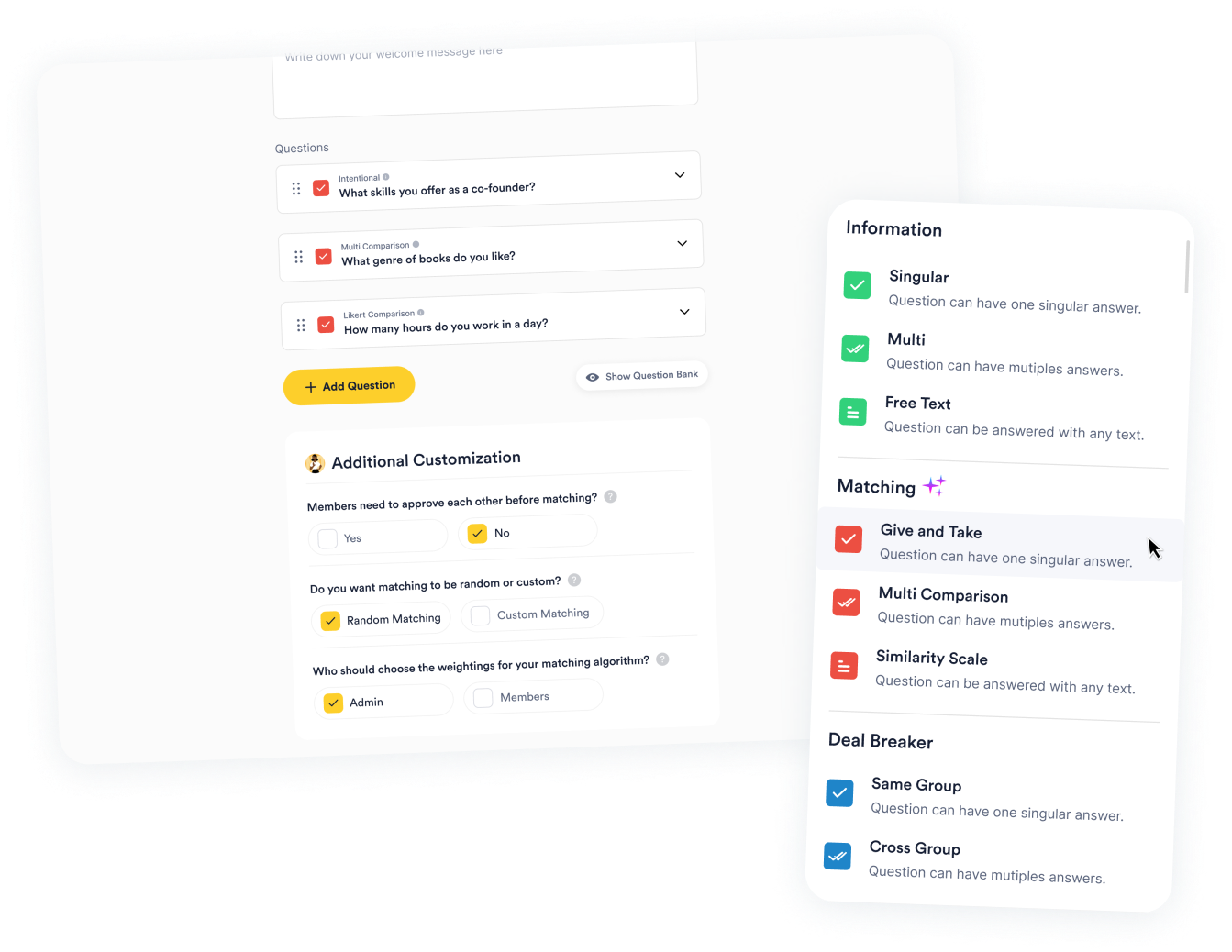
Content Creation
AI has the potential to revolutionize the way in which content is created and disseminated. Through the use of advanced algorithms and machine learning through large and aggregated datasets, AI can assist in the curation and personalization of content, allowing algorithms to tailor content to particular preferences; additionally, AI can also analyze vast amounts of data and generate unique and engaging content that is optimized for the consumer. The applications of AI in the content creation vertical are vast, and perhaps one of the most interesting ways in which AI can impact content creation is through automating certain aspects of the content creation process, enhancing the efficiency and productivity of content creators (allowing them to focus on more strategic and creative tasks). Capsule is a BGV portfolio company that empowers teams to produce high-quality video content for any audience through a variety of AI-powered tools, allowing creators to create content efficiency at scale. Used by prominent companies such as HBO, National Geographic, and TED, Capsule has a suite of AI-powered offerings designed to simplify, streamline, and enhance the video editing and distribution experience; for example, Capsule elucidates the otherwise time-consuming post-production process by using AI-driven templates.
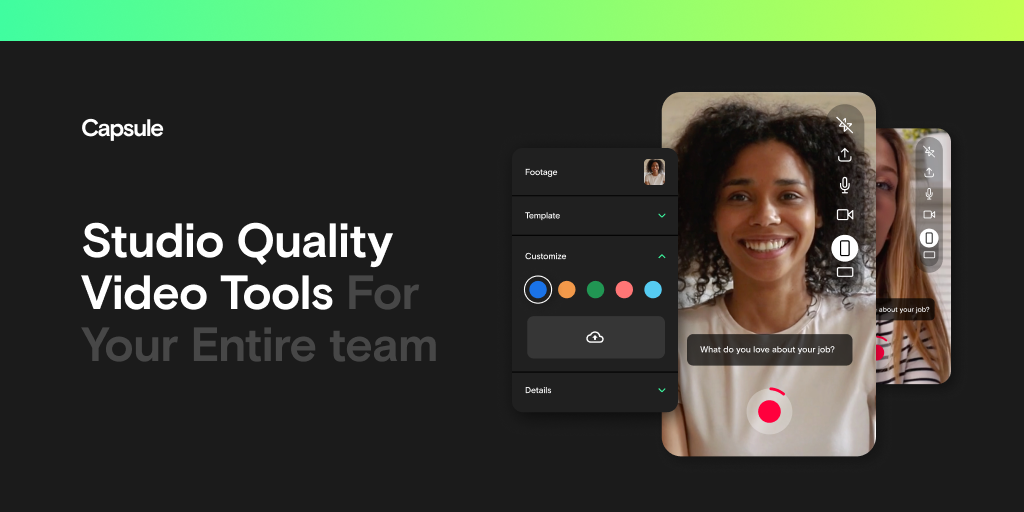
Influencer Marketing
AI can play a substantial role in influencer marketing by analyzing and predicting the effectiveness of different influencers and identifying those who are best suited to represent a particular brand or campaign. Marketing in general has become increasingly data dependent, as platforms such as Facebook and YouTube have used this as a competitive advantage. Through the use of data-driven insights and with the help of reliable predictive analytics, AI can help brands optimize their influencer marketing efforts and maximize their return on ad spend through more intelligent resource allocation. Additionally, AI can assist in the management and optimization of influencer campaigns by automating certain tasks such as tracking performance and identifying opportunities for improvement. Statusphere, one of the first investments out of BGVs Fund II, does all of the above. Statusphere employs AI to upend the traditional influencer marketing landscape, providing a dual-end solution to both influencers and brands. Statusphere pairs content creators with a best-fit brand powered by data and AI, all while allowing for campaigns to be deployed at scale and measured in real time.
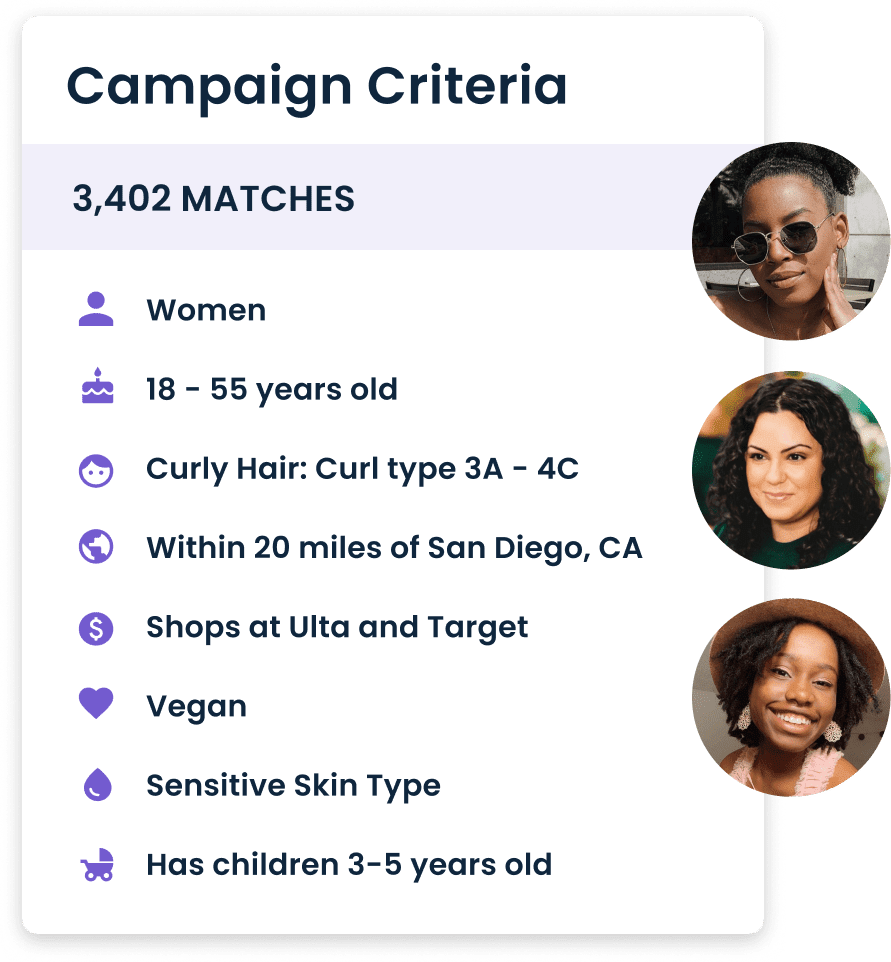
Data Analysis
AI serves a particularly wide field of applications in data analysis. Through verticals such as product management, customer experience, customer research, marketing, and much more, data is seen as a valuable asset in an increasingly-data driven world. AI, being algorithm and model-based, is well-positioned to enhance data aggregation and analysis. This has particular use for organizations that want to extract insights with speed and insight, paving the way for informed decisions to be made with speed. AI has the ability to analyze and process vast amounts of data and identify patterns within those datasets, capturing relationships between data points and trends that would be difficult for humans to discern. At the forefront of this is Viable. Viable automates analysis through powerful and concise reports - saving hundreds of hours by providing natural language reports powered by GPT3, extracting valuable insights that were never before possible.
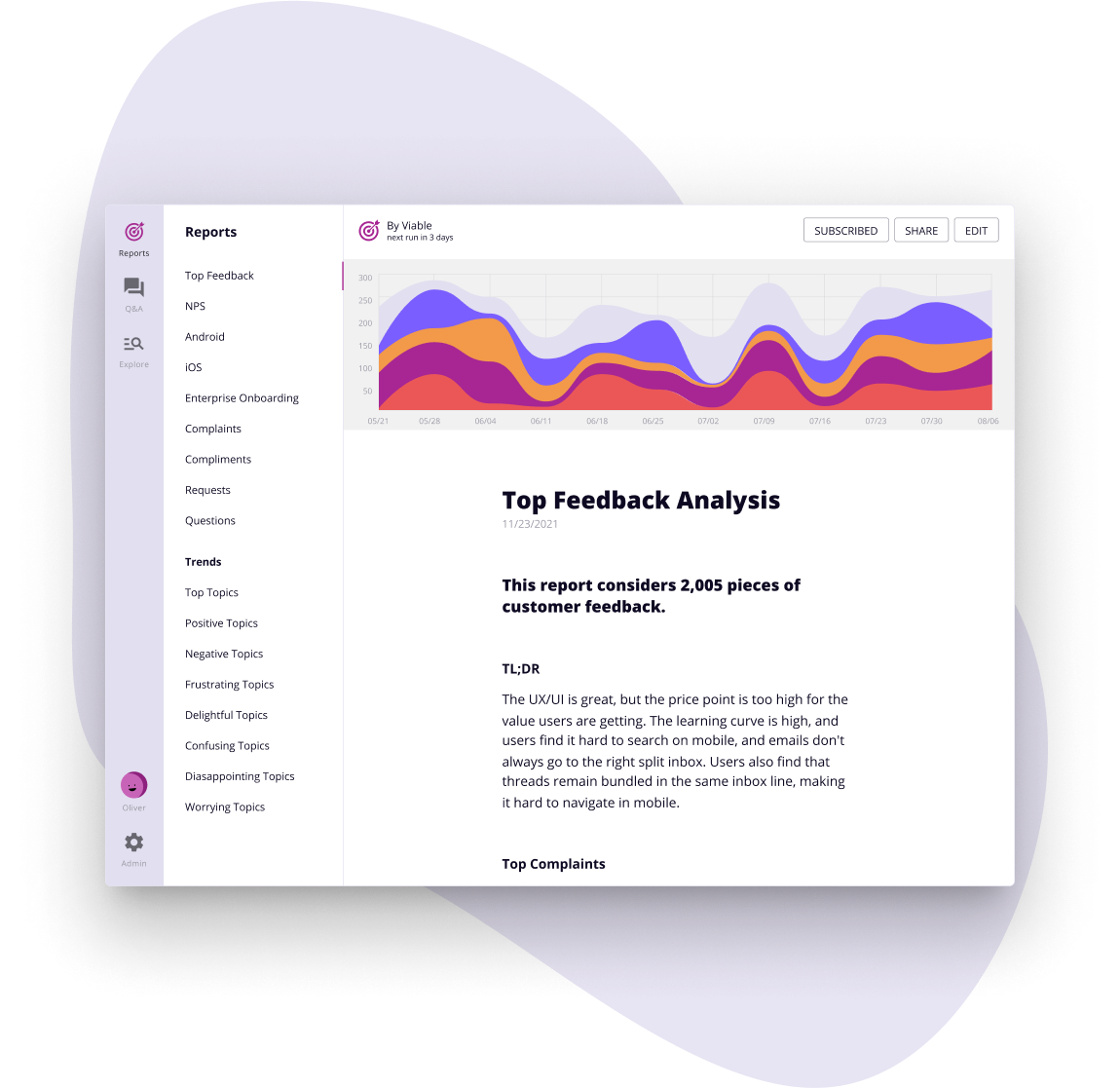
Questions and The Dangers of Modern AI
As the field of AI has developed, so too have the philosophical debates surrounding it. Some of the key philosophical questions that have been raised about AI include: What is consciousness, and can machines truly be conscious? Can machines have emotions and feelings and be considered sentient? What are the ethical implications of creating intelligent machines? Can machines be creative, and if so, what does that mean for the future of human creativity? These questions and many others continue to be explored by philosophers, scientists, and researchers in the field of AI. As technology continues to advance and our understanding of AI deepens, the philosophical debates surrounding this fascinating field are likely to continue for many years to come.
AI, as powerful as it may be, does not come without its potential dangers. One of the critical dangers of AI is the potential for AI-based systems to become too powerful and to potentially even surpass human intelligence. As AI systems become more advanced and are able to perform more complex tasks, there is a risk that by surpassing human intelligence, they can potentially pose a threat to humanity at large. Another danger of AI is the potential for AI systems to be used for malicious or harmful purposes, particularly with respect to algorithmically based AI. For example, AI systems could be used to automate cyberattacks or to create fake news and disinformation, with algorithms potentially being beneficial in perpetuating and spreading this misinformation. Additionally, AI systems could be used to create autonomous weapons that could be lethal even without direct human oversight or control. AI also has the potential for AI systems to be biased or unfair. AI systems, which are generally trained on large datasets, can contain biases and prejudices that are learned and further perpetuated by the AI system. This could lead to unfair and discriminatory outcomes, particularly in fields such as healthcare, finance, and criminal justice.
While AI has the potential to bring many benefits, it also carries some significant dangers and risks. It is important for researchers, policymakers, and society as a whole to carefully consider and address these dangers in order to ensure that AI is developed and used responsibly. Thankfully, there are many organizations that are working to ensure that artificial intelligence (AI) is used responsibly. Some examples of organizations focused on responsible AI include the Partnership on AI, the Future of Life Institute, the Center for Human-Compatible AI, and the AI for Good Foundation. These organizations conduct research, engage with policymakers, and host events focused on AI ethics and responsibility. The field of AI ethics and responsibility is constantly evolving and growing, and there are many other organizations and initiatives focused on ensuring that while AI should be allowed to prosper and deliver value, its potential downsides need to be considered when developing it.